Chapter 13. Statistical Estimation
Higher category: 【Statistics】 Statistics Overview
1. overview
1. overview
⑴ statistical estimation: estimating the characteristics of a population through samples
⑵ state space: ℝn. a set of all the observed samples
2. point estimation (parametric approach, location type)
⑴ definition: estimating parameters from samples (x1, ···, xn)

① parameter: values showing the characteristics of the population. μ, σ, θ, λ, etc
② μ: mean of population
③ σ: standard deviation of population
④ θ: θ: probability of success in Bernoulli distribution or binomial distribution
⑤ λ: λ of Poisson distribution or exponential distribution
⑵ sampling distribution (empirical distribution)
⑶ point estimator: for parameter θ,
① definition 1. point estimator is not a single number but a function
② definition 2. point estimator is a function for X1, ···, Xn
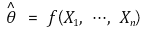
③ definition 3. the probability of a point estimator is a function of θ
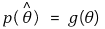
⑷ criteria for a good point estimator
① expected error or mean squared error (MSE): also called model risk
○ bias-variance decomposition
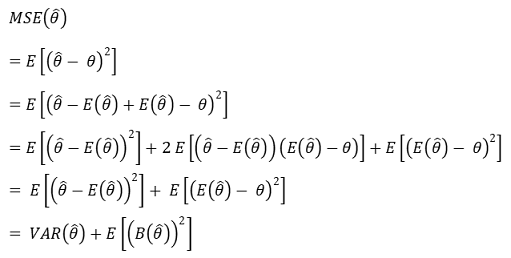
○ as the covariance of bias and chance error is 0 intuitively, we can remove the intermediate term
○ the strategy to reduce the bias increases the model variance
○ the strategy to reduce the model variance increases the bias
② criterion 1. bias: also called systemic error, non-random error, and model bias
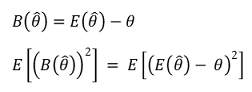
○ small bias required
○ cause: underfitting, lack of domain knowledge
○ solutions: use of more complex models, use of models suitable for domain
○ unbiased estimator: B = 0 ⇔ sample mean = population mean. if there’s unbiasedness, it’s a good estimator
○ example 1. sample mean: unbiased estimator of population mean
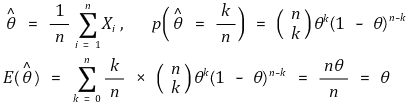
○ example 2. sample variance: unbiased estimator of population variance
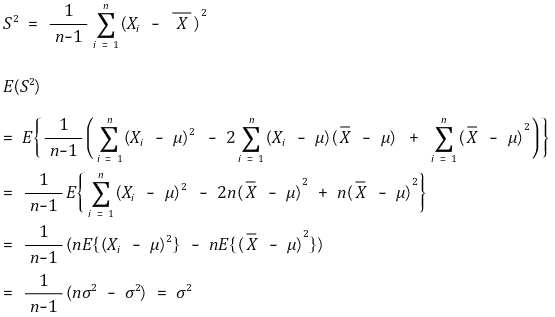
○ example 3. sample covariance

○ example 4. when Xi ~ u[0, θ], either unbiased estimator or not
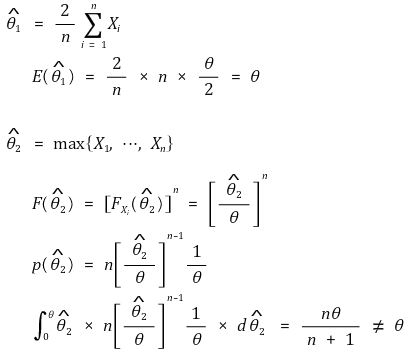
③ criterion 2. efficiency: related to random chance error
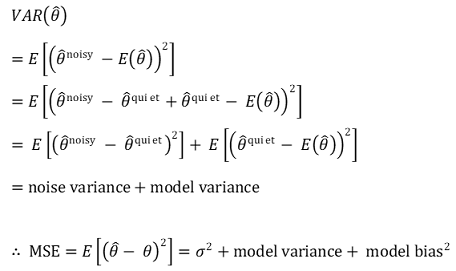
○ small variance is required based on the premise of unbiased estimator
○ 2-1. noise variance: also called 1st chance error and observation variance. marked as σ2
○ example: error of the instrument itself, noise of the target itself
○ there are attempts to measure these information, but there are many difficulties
○ 2-2. model variance: also called 2nd chance error
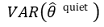
○ chance error due to the fact that the sample group is a randomly extracted set from the population
○ cause: overfitting
○ solution: using a simpler model
○ bias-variance tradeoff: as model complexity increases, the bias decrease, but model variance increases, resulting in trade-off relationship and optimal complexity
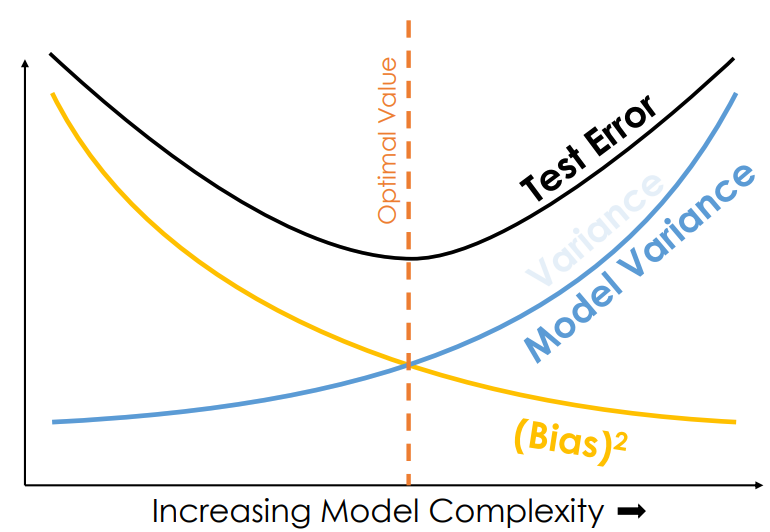
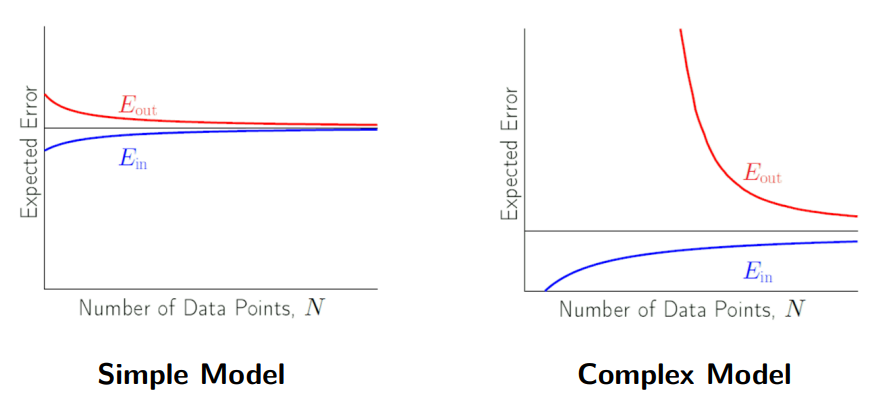
○ BLUE (best linear unbiased estimator): the estimator of the smallest variance among linear unbiased estimators
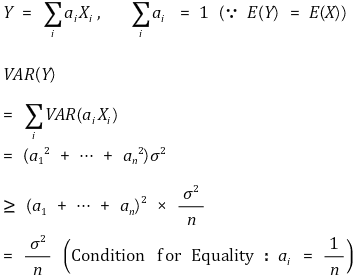
○ uniformly minimum variance unbiased estimator (UMVU)
○ definition: the estimator of the smallest variance among unbiased estimators including non-linear unbiased estimators
○ the direct calculation of Fisher information In
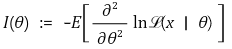
○ the indirect calculation of Fisher information In
○ Cramér–Rao lower bound = 1 / In
○ if an estimator is equal to the Cramér–Rao lower bound, it is UMVU
○ Example 1. Sample Mean and Sample Median
○ Asymptotic distribution of the sample mean when the population distribution is normal
○ Asymptotic distribution of the sample median when the population distribution is normal
○ When the population distribution is normal, the Hodges–Lehmann estimator, defined as median[(Xi + Xj) / 2 : i ≤ j], is more efficient than the sample mean.
○ If the random variable follows a double-exponential distribution, the sample mean has greater variance than the sample median.
○ Example 2. Sample Standard Deviation (Sn) and MAD (Median Absolute Deviation)
○ Sn →d σ
○ MAD = median( X1 - μ , ⋯, Xn - μ ) →d Φ-1(3/4) σ = 0.676 σ
④ criterion 3. consistency and consistent estimator
○ asymptotic property: characteristic of sample, of which size is approaching ∞
○ asymptotic unbiasedness: the case in which the unbiasedness is established when n → ∞. it is related to the law of large numbers
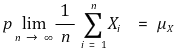
○ asymptotic efficiency

○ consistency: the property of the estimator converging into a parameter

○ X is a random variable, but generally considered as a specific constant
○ example: the following is a poor random variable because it is inconsistent

○ ARE (Asymptotic Relative Efficiency): The ratio of the asymptotic variances of two random variables. A significant deviation from the theoretical value may suggest the presence of outliers.
⑤ Criterion 4. Robust Estimator: Investigates whether the estimator is more sensitive to outliers.
⑥ Criterion 5. Least Squared Estimator
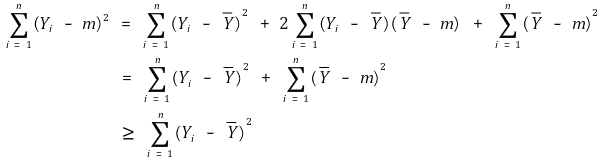
⑸ method 1. discrete probability distribution and maximum probability
① it uses the definition of binomial coefficients
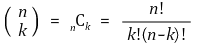
② example
○ situation: number of members of a population are estimated through marking-and-recapture method
○ given the number of members N, the number of firstly captured members m, the number of lastly captured members n, the number of lastly marked memebers x
○ probability distribution: hypergeometric distribution
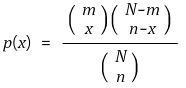
○ question: the most reasonable value of N
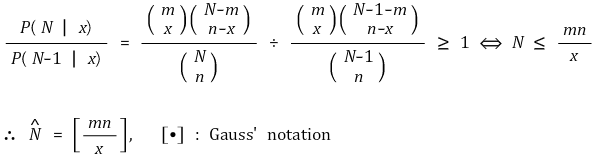
⑹ method 2. method of moment estimator (MOM): also called sample analog estimation
① definition: the method of calculating the estimator of θ in a way of θˆ =g-1((1/n) × ∑Xik) based on the fact that E(Xk) = g(θ) ⇔ θ = g-1(E(Xk))
○ E(Xk): moment or population moment
○ (1/n) × ∑Xik: sample moment
○ the moment is a constant, and the sample moment is a random variable with a constant distribution
○ consistency: by the law of large numbers, the sample moment converges into the moment
② sample moment
○ k-th order sample moment for origin
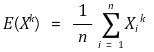
○ k-th order sample moment for sample mean

③ example

⑺ method 3. maximum likelihood method (ML)
① definition
○ θ: parameter
○ θ*: the estimator of the parameter θ
○ θML: the maximum likelihood estimator of the parameter θ
② likelihood: the possibility of something happening
③ likelihood function
○ the probability that a given sample will come out when θ* is given.
○ also known as product of likelihoods
○ that is, p(X | θ*)
○ marked as ℒ
④ log likelihood function: taking a log into the likelihood function
○ marked as ℓ = ln ℒ
⑤ maximum likelihood estimation: examining θML that maximizes the likelihood function p(X | θ)

○ assumption : the closer the θ* is to parameter θ, the greater the likelihood function will be
○ 1st. differentiation of log likelihood function: acquire θ* that makes the local maximum on a valid interval
○ 2nd. if the local maximum exists: it is assumed that the θ* that makes the local maximum is θML
○ 3rd. if the local maximum doesn’t exist: it is assumed that θ* with higher likelihood of both ends of a valid interval is θML
○ maximum likelihood estimation and Hessian matrix: a useful method for obtaining estimators of all differentiable functions
○ step 1. get the second order approximation by obtaining the Taylor series for θk , and calculate the solution θk+1 = θk + dk that maximize the approximated equation
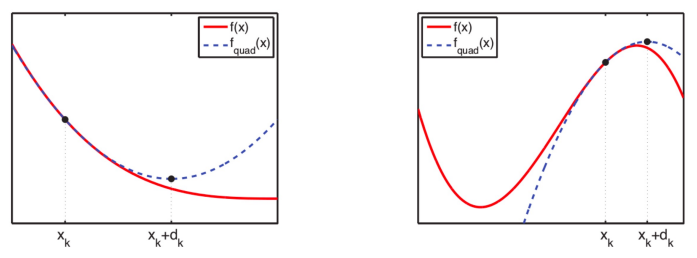
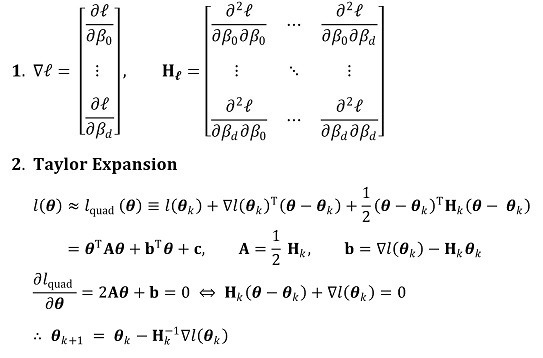
○ step 2. Newton-Raphson method: updating θk will eventually reach the global maximum
○ example: logistic regression
⑥ Maximum likelihood estimator: when sample X is given, the function G corresponding to θML that maximizes the likelihood
○ θML = Gℓ (ℓ) = Gℒ(ℒ)
○ limitation of the estimator: there are limits on the assumption of maximum likelihood estimation
○ the estimator favored by statisticians.
⑦ MAP(maximum a posteriori)
○ The maximum likelihood estimation is a special example of Bayes rule
○ MLE assumes a uniform distribution over the parameters, but when a prior distribution is available, the MAP method is used.
⑧ Example 1.
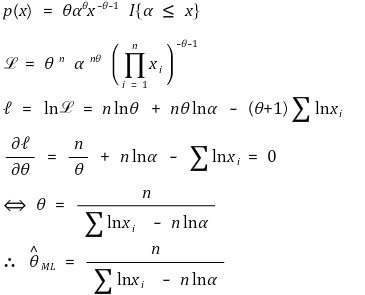
⑨ Example 2.
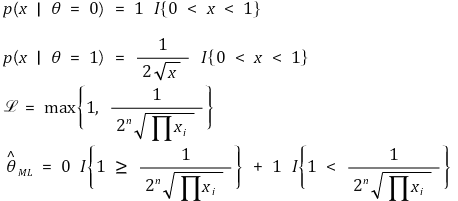
⑩ Example 3.
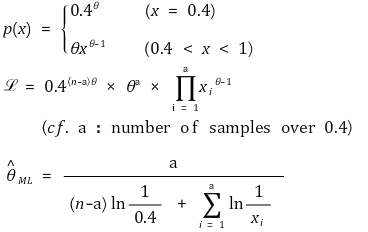
⑪ Example 4. the maximum likelihood estimation might not be determined solely

⑫ Characteristic 1. consistency
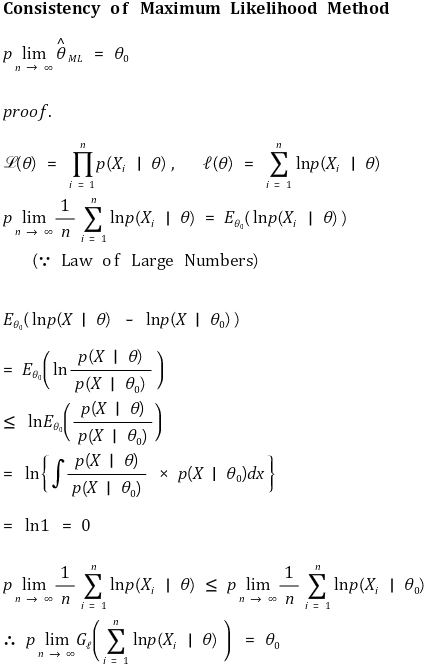
⑬ Characteristic 2. asymptotic normal distribution
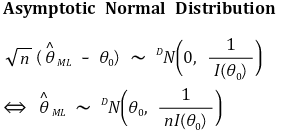
⑭ Characteristic 3. invariance: if θML is the maximum likelihood estimator of θ, g(θML) is the maximum likelihood estimator of g(θ)
3. interval estimation (scaling type)
⑴ definition: estimating which interval the parameter is in through the samples
① purpose of introduction: the probability that the point estimator exactly matches the actual parameter is zero
② confidence level (confidence coefficient)
○ P(θleft < θ < θright) = 1 - α, 0 < α < 1
○ threshold: values that constitute the boundary of the confidence interval. θleft, , θright, etc
○ 1 - α: confidence level (confidence coefficient)
○ α: rejection probability or significance level
○ confidence interval: the interval [θleft, θright] , the probability of θ being on which is (1 - α) × 100%
③ notes
○ P(Z > 1.65) = 5% ⇔ P( Z > 1.65) = 10%
○ P(Z > 1.96) = 2.5% ⇔ P( Z > 1.96) = 5%
○ P(Z > 2.58) = 0.5% ⇔ P( Z > 2.58) = 1%
④ 68 - 95 - 99.7 rule
○ μ ± 1 × σ: 68.27 %
○ μ ± 2 × σ: 95.45 %
○ μ ± 3 × σ: 99.73 %
⑵ case 1. when Xi ~ N(μ, σ2) and the population variance σ2 is known
① overview: a normal distribution is used
② method
○ introduction: when μ is known, the probability of Xavg (confidence level: α) is as follows
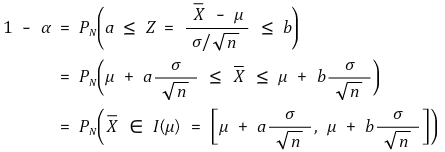
○ change of ideas: Xavg ∈ I (μ) ⇔ μ ∈I (Xavg) (confidence level: α)
○ meaning: it means the probability distribution of μ when Xavg is known
○ it is noted that the probability distribution of μ follows the same conceptual framework of the probability distribution of Xavg when μ is known
○ draw your own picture to confirm
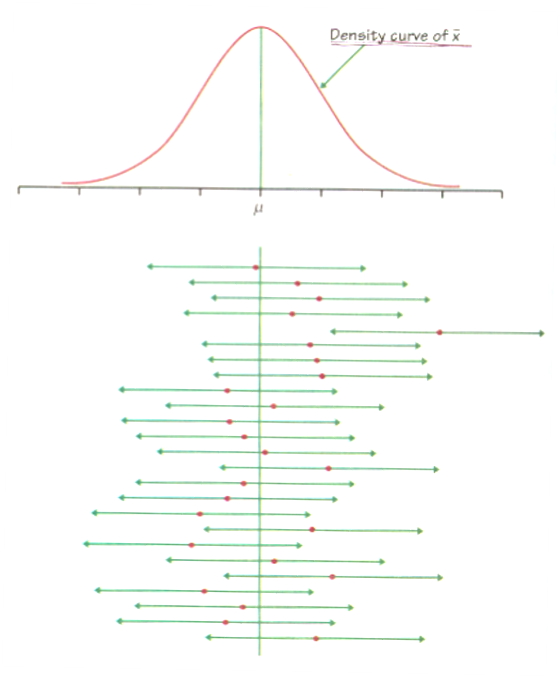
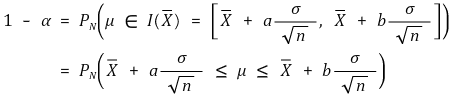
○ pivotal estimation: for the shortest confidence interval, it should be established that |a| = |b|, i.e. a = -zα/2, b = zα/2. here, the proof is omitted

③ if you know the distribution function
○ example 1. F(x) = √x / θ, 0 ≤ x ≤ θ2: the 90% confidence interval is as follows

○ example 2. F(x) = (x / θ)n: the 90% confidence interval is as follows

⑶ case 2. when Xi ~ N(μ, σ2) and the population variance σ2 is unknown
① overview
○ normal distribution needs to know the variance of the population
○ in reality, the sample variance is used because the population variance is unknown
○ the distribution of sample mean when using sample variance instead of population variance is exactly t-distribution
② example 1. sample mean
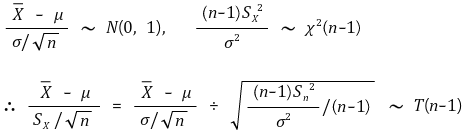
○ introduction: when μ is known, the probability of Xavg (confidence level: α)
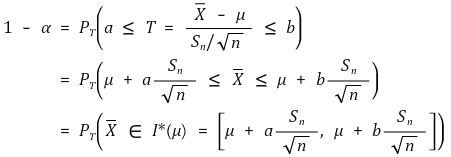
○ change of ideas: Xavg ∈ I* (μ) ⇔ μ ∈ I* (Xavg) (confidence level: α)
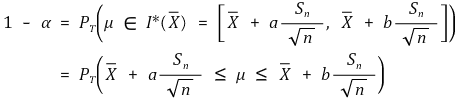
○ pivotal estimation: for the shortest confidence interval, it should be established that |a| = |b|, i.e. a = - tα/2, b = tα/2. here, the proof is omitted

③ example 2. (case 1) when Xi (μX, σ2) (i = 1, ···, n) and Yj (μY, σ2) (i = 1, ···, n) are paired
○ also called paired estimation (matched sample estimation)
○ in fact, there is only one variable: after defining Wi = Xi - Yi, manipulate example 1

○ an example of a paired sample
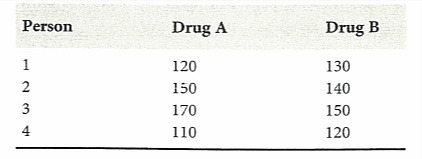
○ an example of an independent sample
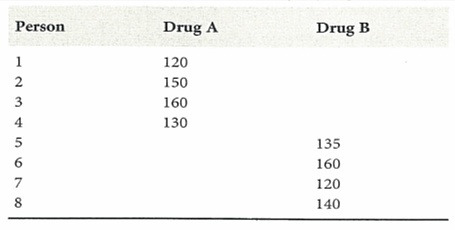
④ example 3. (case 2) difference of two sample means: when Xi (μX, σ2) (i = 1, ···, n) and Yj (μY, σ2) (j = 1, ···, m) are independent
○ when the variances of two sample means are same in unpaired sample estimation (pooled sample estimation)
○ formula
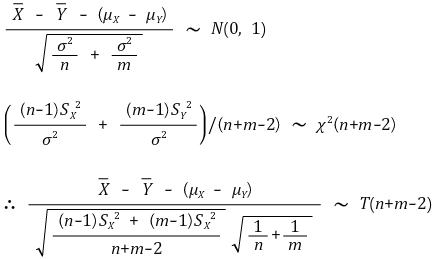
○ confidence interval for confidence level α

⑤ example 4. (case 3) difference of sample means: when Xi (μX, σX2) (i = 1, ···, n) and Yj (μY, σY2) (j = 1, ···, m) are independent (assuming σX ≠ σY)
○ when the variances of two sample means are different in unpaired sample estimation (pooled sample estimation)
○ Welch approach is used
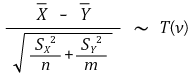
○ degree of freedom in (case 3) is lower than (case 2) → power of test decreases
○ the formula of ν is very complex
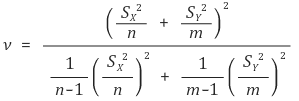
⑥ example 5. confidence interval of population variance
○ note that there is no obvious solution in minimizing the size of the confidence interval: numerical analysis should be used
○ the given model
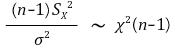
○ confidence interval for confidence level α
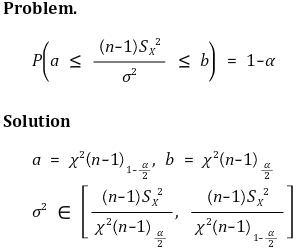
⑦ example 6. ratio of population variance
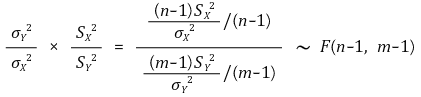
○ confidence interval for confidence level α
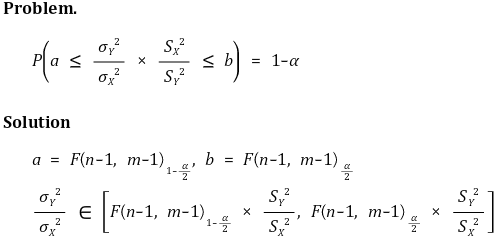
⑷ case 3. when samples do not follow normal distribution, but there are many samples
① central limit theorem: if n is large enough, the distribution of sample mean converges into normal distribution
○ formula
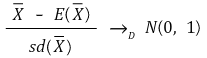
○ t distribution eventually converges into normal distribution
② number of samples
○ normality is typically achieved with only 25 ~ 30 samples
○ for a symmetric unimodal distribution (having one extreme value), n = 5 is sufficient
③ example 1. population ratio
○ given model
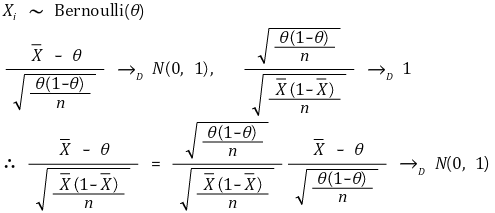
○ confidence interval for confidence level α

④ example 2. correlation coefficient
○ null hypothesis H0: correlation coefficient = 0
○ alternative hypothesis H1: correlation ceofficient ≠ 0
○ calculation of t statistics: for the correlation coefficient r obtained from the sample,
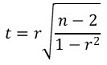
○ the above statistic follows the student t distribution with a degree of freedom of n - 2 (assuming the number of samples is n)
Input : 2019.06.19 14:23