Chapter 9. Theorems of Statistics I
Higher category: 【Statistics】 Statistics Overview
9. Laplace’s rule of succession
1. Markov inequality
⑴ theorem
when X is the random variable and a > 0, the following is established:

⑵ proof 1.
let x1, ···, xn be events that make up the random variable X. by reordering the events, we can acquire | xi | ≥ a ⇔ i = r+1, ···, n
⑶ proof 2.
2. Chebyshev’s inequality
⑴ theorem
there is a random variable with expected value of m and variance of σ2. the variance is a finite value. then, for any real number k > 0, the following inequality is established:

however, meaningful information is provided only when k>1.
⑵ proof 1.
let x1, ···, xn be events that make up the random variable X. by rearranging the above events, we can acquire | xi - m | ≥ kσ ⇔ i = r+1, ···, n.
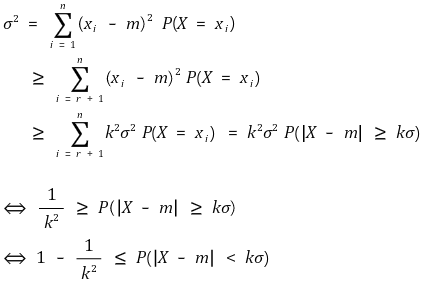
⑶ proof 2.
the case of Markov inequality with k = 2, ε = kσ, X → X - μX is Chebyshev’s inequality
3. Cantelli inequality (one-tailed chebyshev inequality)
⑴ theorem
there is a random variable with expected value of m and variance of σ2. the variance is a finite value. then, for any real number k > 0, the following inequality is established:

⑵ proof
① m = 0
let x1, ···, xn be events that make up the random variable X. by reordering the events, we can acquire xi < kσ ⇔ i = r+1, ···, n. if we put kσ = t,
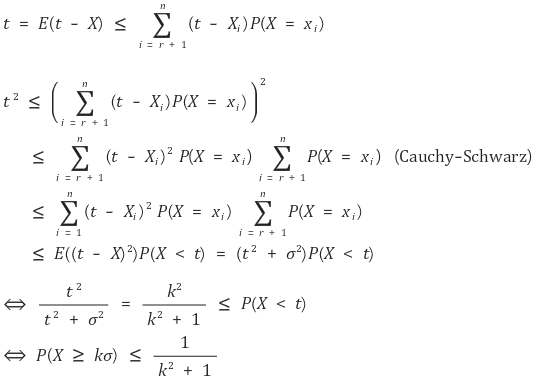
② m = μ ≠ 0
X’ = X - μ is a random variable with an expected value of 0 and a variance of σ2. so the following is established:

4. Cauchy-Schwarz inequality
⑴ theorem

⑵ proof
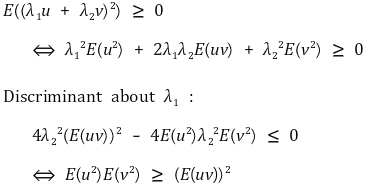
⑶ example
① E(1 / X) ≥ 1 / E(X)
② ln (E(X)) ≥ E(ln X)
5. Jensen’s inequality
⑴ convex function and concave function
① convex set: it refers to the case where the internally dividing points of any two points in a set are always elements of the set
② convex function: the function where {(x, y) | y ≥ f(x)} is a convex set. it is similar to ‘convex downward’
③ strictly convex function
④ concave function: the function where{(x, y) | y ≤ f(x)}is a convex set. it is similar to ‘convex upward’
⑤ strictly concave function
⑥ linear function: the case of both covex function and concave function
⑵ relationship between convexity and the secondary derivative: f”(x) ≥ 0 and the convex function are necessary and sufficient. f”(x) ≤ 0 and the concave function are necessary and sufficient
① proof 1.
② proof 2. an easy proof showing that for the convex function, f”(x) ≥ 0 is established and for concave function, f”(x) ≤ 0 is established
⑶ Jensen’s inequality: expansion of dimension
① when f is a convex function, the following inequality is established:
② when f is a concave function, the following inequality is established:
③ proof 1. mathematical induction
○ proof
Assuming that f is a convex function, the following inductive hypothesis holds.
Similarly, in the case where f is a concave function, the inductive hypothesis can also be shown to hold. The bivariate case has already been proven above.
④ proof 2. introduction of a linear function.
○ proof
let’s define ℓ(x) as a straight-line function that passes (E(X), f(E(X)), and tangles f(x). let’s say f is a convex function. then,
⑷ economic statistical meaning.
① risk-loving
○ convex function
○ if the utility function for the mean is smaller
○ if you prefer adventure

② risk-avoiding
○ concave function
○ if the utility function for the mean is greater
○ if you prefer predictable results

③ risk-neutral

6. law of large numbers (LLN)
⑴ theorem
for any real number α > 0, the following equation is established:

⑵ proof
suppose the mean of the random variable X is m, and the standard deviation of it is σ. for any real number k > 0, let’s apply Chebyshev inequality
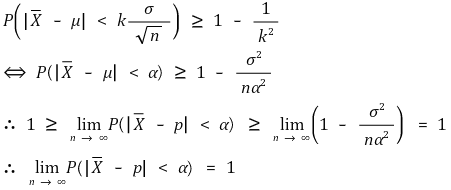
⑶ sufficient condition
① the random variable Xi, i = 1, ···, n is i.i.d
② VAR(Xi) < ∞
⑷ Application
① Weak Law of Large Numbers (Weak LLN, WLLN)
○ Convergence in probability
○ Guarantees that the probability of the sample mean approaching the expected value converges to 1.
○ In other words, it only ensures that the likelihood of the sample mean getting close to the expected value increases in a probabilistic sense.
② Strong Law of Large Numbers (Strong LLN, SLLN)
○ Convergence almost surely: If this holds, convergence in probability naturally follows.
○ Guarantees that in almost all cases, the sample mean will certainly converge to the expected value.
○ In other words, even when looking at individual realizations, it ensures that the mean ultimately converges to the expected value.
7. central limit theorem (CLT)
⑴ outline
① definition: when the number of samples is infinitely large, the sample sum and sample mean are normally distributed regardless of the distribution of the samples
② mathematical expression: uses convergence in distribution
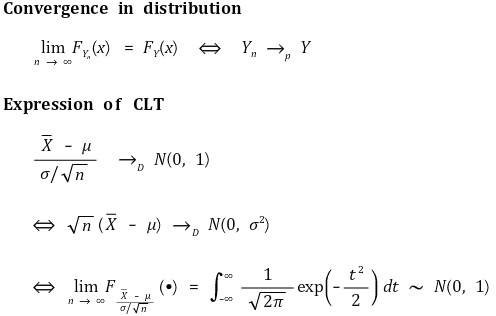
⑵ Proof 1.
if the probability of an event occurring is p, the probability of an event occurring k times during n trials is as follows:

as n approaches infinity, this probability distribution changes almost continuously. therefore, we can think of the following function, which takes the real value as the domain of x

① average
if f has the maximum value at the point of x = m in the distribution, the following equation is established:

pay attention to the next

however, the Sterling approximation can strictly prove that the definition of n! is as follows
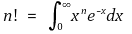
therefore
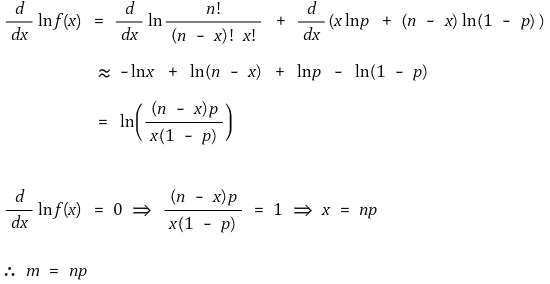
② normal distribution approximation of the binomial distribution
for Δx = x - m that is small enough, the following holds true
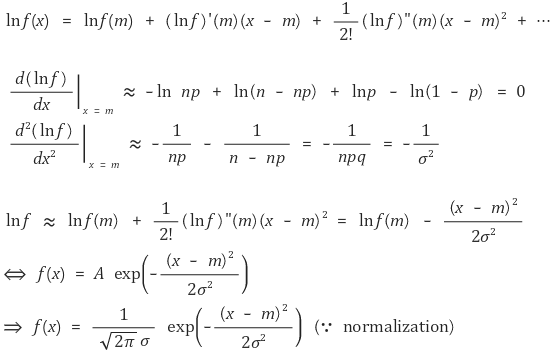
as a result, B(n, p) is close to N(np, npq) around the average (assuming, n ≫ 1)
⑶ proof 2. introduction of m(t) and moment generating function
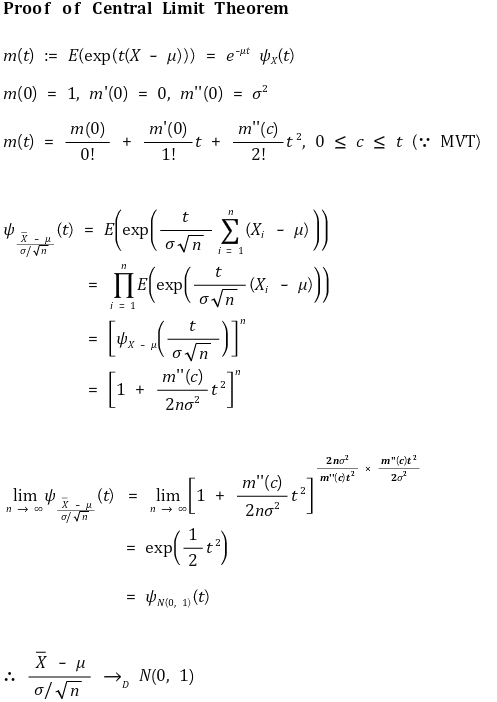
⑷ sufficient condition
① Xi, i = 1, ···, n is i.i.d
② 0 < VAR(Xi) < ∞
⑸ Application
① Conditions for Normal Approximation of Binomial Distribution: Variance = npq ≥ 10
② Conditions for Normal Approximation of Poisson Distribution: λ ≥ 30
⑹ Example problems for central limit theorem
8. Slutsky’s theorem
⑴ convergence in probability: for random variables S1, S2, ···, Sn,
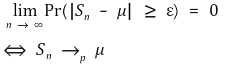
⑵ convergence in distribution: for random variables S1, S2, ···, Sn, for each distribution function F1, F2, ···, Fn, and for S that takes F as its distribution function,
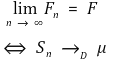
⑶ Slutsky’s theorem (algebra of probability limit): when there exist p lim Xn, p lim Yn,
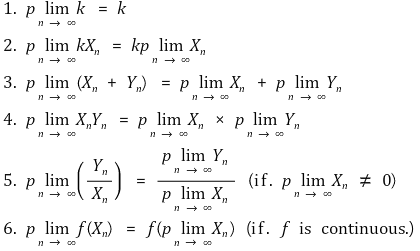
① the above 6-th formula is called CMT (continuous mapping theorem)
9. Laplace’s rule of succession
⑴ definition: when k positive results are obtained during n trials, the probability of the positive result in the n+1-th trial is as follows
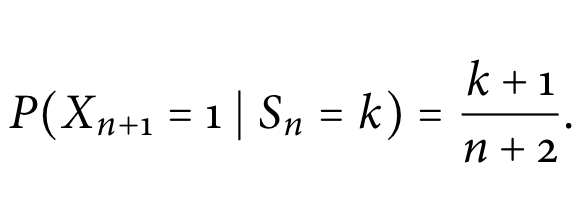
⑵ proof (refhttps://jonathanweisberg.org/pdf/inductive-logic-2.pdf)
Input : 2019.05.03 15:02